注重体验与质量的电子书资源下载网站
分类于: 人工智能 职场办公
简介
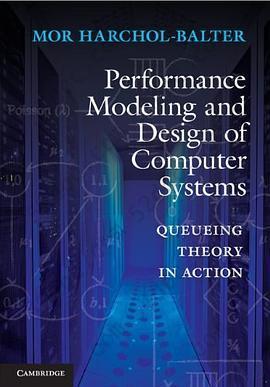
Performance Modeling and Design of Computer Systems: Queueing Theory in Action 豆 0.0分
资源最后更新于 2020-08-19 16:12:55
作者:Mor Harchol-Balter
出版社:Cambridge University Press
出版日期:2013-01
ISBN:9781107027503
文件格式: pdf
标签: 计算机 Performance 数学 Queueing 计算机科学 排队论 Systems 性能
简介· · · · · ·
Tackling the questions that systems designers care about, this book brings queueing theory decisively back to computer science. The book is written with computer scientists and engineers in mind and is full of examples from computer systems, as well as manufacturing and operations research. Fun and readable, the book is highly approachable, even for undergraduates, while still ...
目录
Table of Contents
Part I: Introduction to Queueing
1 Motivating Examples of the Power of Analytical Modeling
1.1 What is Queueing Theory?
1.2 Examples of the Power of Queueing Theory
2 Queueing Theory Terminology
2.1 Where We Are Heading
2.2 The Single-Server Network
2.3 Classification of Queueing Networks
2.4 Open Networks
2.5 More Metrics: Throughput and Utilization
2.6 Closed Networks
2.6.1 Interactive (Terminal-Driven) System
2.6.2 Batch Systems
2.6.3 Throughput in a Closed System
2.7 Differences between Closed and Open Networks
2.7.1 A Question on Modeling
2.8 Related Readings
2.9 Exercises
Part II: Necessary Probability Background
3 Probability Review
3.1 Sample Space and Events
3.2 Probability Defined on Events
3.3 Conditional Probabilities on Events
3.4 Independent Events and Conditionally Independent Events
3.5 Law of Total Probability
3.6 Bayes Law
3.7 Discrete versus Continuous Random Variables
3.8 Probabilities and Densities
3.8.1 Discrete: Probability Mass Function
3.8.2 Continuous: Probability Density Function
3.9 Expectation and Variance
3.10 Joint Probabilities and Independence
3.11 Conditional Probabilities and Expectations
3.12 Probabilities and Expectations via Conditioning
3.13 Linearity of Expectation
3.14 Normal Distribution
3.14.1 Linear Transformation Property
3.14.2 Central Limit Theorem
3.15 Sum of a Random Number of Random Variables
3.16 Exercises
4 Generating Random Variables
4.1 Inverse Transform Method
4.1.1 The Continuous Case
4.1.2 The Discrete Case
4.2 Accept/Reject Method
4.2.1 Discrete Case
4.2.2 Continuous Case
4.2.3 Some Hard Problems
4.3 Readings
4.4 Exercises
5 Sample Paths, Convergence, and Averages
5.1 Convergence
5.2 Strong and Weak Laws of Large Numbers
5.3 Time Average versus Ensemble Average
5.3.1 Motivation
5.3.2 Definition
5.3.3 Interpretation
5.3.4 Equivalence
5.3.5 Simulation
5.3.6 Average Time in System
5.4 Related readings
5.5 Exercises
Part III: The Predictive Power of Simple Operational Laws: What-If Questions and Answers
6 Little's Law and Other Operational Laws
6.1 Little's Law for Open Systems
6.2 Intuitions
6.3 Little's Law for Closed Systems
6.4 Open Systems
6.4.1 Statement via Time Averages
6.4.2 Proof
6.4.3 Corollaries
6.5 Closed Systems
6.5.1 Statement via Time Averages
6.5.2 Proof
6.6 Generalized Littleās Law
6.7 Examples Applying Little's Law
6.8 More Operational Laws: The Forced Flow Law
6.9 Combining Operational Laws
6.10 Device Demands
6.11 Readings and Further Topics Related to Little's Law
6.12 Exercises
7 Modification Analysis: What-if for Closed Systems
7.1 Review
7.2 Asymptotic Bounds for Closed Systems
7.3 Modification Analysis for Closed Systems
7.4 More Modification Analysis Examples
7.5 Comparison of Closed and Open Networks
7.6 Readings
7.7 Exercises
Part IV: From Markov Chains to Simple Queues
8 Discrete-Time Markov Chains
8.1 Discrete-Time versus Continuous-Time Markov Chains
8.2 Definition of a DTMC
8.3 Examples of Finite-State DTMCs
8.3.1 Repair Facility Problem
8.3.2 Umbrella Problem
8.3.3 Program Analysis Problem
8.4 Powers of P: n-Step Transition Probabilities
8.5 Stationary Equations
8.6 The Stationary Distribution Equals the Limiting Distribution
8.7 Examples of Solving Stationary Equations
8.7.1 Repair Facility Problem with Cost
8.7.2 Umbrella Problem
8.8 Infinite-State DTMCs
8.9 Infinite-State Stationarity Result
8.10 Solving Stationary Equations in Infinite-State DTMCs
8.11 Exercises
9 Ergodicity Theory
9.1 Ergodicity Questions
9.2 Finite-State DTMCs
9.2.1 Existence of the Limiting Distribution
9.2.2 Mean Time between Visits to a State
9.2.3 Time Averages
9.3 Infinite-State Markov Chains
9.3.1 Recurrent versus Transient
9.3.2 Infinite Random Walk Example
9.3.3 Positive Recurrent versus Null Recurrent
9.3.4 Relating Limiting Probabilities to Stationary Probabilities
9.4 Ergodic Theorem of Markov Chains
9.5 Time Averages
9.6 Limiting Probabilities Interpreted as Rates
9.7 Time-Reversibility Theorem
9.8 When Chains are Periodic or not Irreducible
9.8.1 Periodic Chains
9.8.2 Chains that are not Irreducible
9.9 Conclusion
9.10 Proof of Ergodic Theorem of Markov Chains
9.11 Exercises
10 Real-World Examples: Google, Aloha, and Harder Chains
10.1 Google's PageRank algorithm
10.1.1 Google's DTMC Algorithm
10.1.2 Problems with Real Web Graphs
10.1.3 Googleās Solution to Dead Ends and Spider Traps
10.1.4 Evaluation of the PageRank Algorithm
10.1.5 Practical Implementation Considerations
10.2 Aloha Protocol Analysis
10.2.1 The Slotted Aloha Protocol
10.2.2 The Aloha Markov Chain
10.2.3 Properties of the Aloha Markov Chain
10.2.4 Improving the Aloha Protocol
10.3 Generating Functions for Harder Markov Chains
10.3.1 The z-Transform
10.3.2 Solving the Chain
10.4 Readings and Summary
10.5 Exercises
11 Exponential Distribution and the Poisson Process
11.1 Definition of the Exponential Distribution
11.2 Memoryless Property of the Exponential
11.3 Relating Exponential to Geometric
11.4 More Properties of the Exponential
11.5 The Celebrated Poisson Process
11.6 Merging Independent Poisson Processes
11.7 Poisson Splitting
11.8 Uniformity
11.9 Exercises
12 Transition to Continuous-Time Markov Chains
12.1 Defining CTMCs
12.2 Solving CTMCs
12.3 Generalization and Interpretation
12.3.1 Interpreting the Balance Equations for the CTMC
12.3.2 Summary Theorem for CTMCs
12.4 Exercises
13 M/M/1 and PASTA
13.1 The M/M/1 Queue
13.2 Examples Using an M/M/1 Queue
13.3 PASTA
13.4 Further Reading
13.5 Exercises
Part V: Server Farms and Networks: Multi-Server, Multi-Queue Systems
14 Server Farms: M/M/k and M/M/k/k
14.1 Time-Reversibility for CTMCs
14.2 M/M/k/k Loss System
14.3 M/M/k
14.4 Comparison of Three Server Organizations
14.5 Readings
14.6 Exercises
15 Capacity Provisioning for Server Farms
15.1 What Does Load Really Mean in an M/M/k?
15.2 The M/M/1
15.2.1 Analysis of the M/M/1
15.2.2 A First Cut at a Capacity Provisioning Rule for the M/M/k
15.3 Square-Root Staffing
15.4 Readings
15.5 Exercises
16 Time-Reversibility and Burke's Theorem
16.1 More Examples of Finite-State CTMCs
16.1.1 Networks with Finite Buffer Space
16.1.2 Batch System with M/M/2 I/O
16.2 The Reverse Chain
16.3 Burkeās Theorem
16.4 An Alternative (Partial) Proof of Burke's Theorem
16.5 Application: Tandem Servers
16.6 General Acyclic Networks with Probabilistic Routing
16.7 Readings
16.8 Exercises
17 Networks of Queues and Jackson Product Form
17.1 Jackson Network Definition
17.2 The Arrival Process into Each Server
17.3 Solving the Jackson Network
17.4 The Local Balance Approach
17.5 Readings
17.6 Exercises
18 Classed Network of Queues
18.1 Overview
18.2 Motivation for Classed Networks
18.3 Notation and Modeling for Classed Jackson Networks
18.4 A Single-Server Classed Network
18.5 Product Form Theorems
18.6 Examples Using Classed Networks
18.6.1 Connection-Oriented Network Example
18.6.2 Distribution of Job Classes Example
18.6.3 CPU-Bound and I/O-Bound Jobs Example
18.7 Readings
18.8 Exercises
19 Closed Networks of Queues
19.1 Motivation
19.2 Product-Form Solution
19.2.1 Local Balance Equations for Closed Networks
19.2.2 Example of Deriving Limiting Probabilities
19.3 Mean Value Analysis (MVA)
19.3.1 The Arrival Theorem:
19.3.2 Iterative Derivation of Mean Response Time
19.3.3 An MVA Example
19.4 Readings
19.5 Exercises
Part VI: Real-WorldWorkloads: High Variability and Heavy Tails
20 Tales of Tails: Real-World Workloads
20.1 Grad School Tales ... Process Migration
20.2 UNIX Process Lifetime Measurements
20.3 Properties of the Pareto Distribution
20.4 The Bounded Pareto distribution
20.5 Heavy Tails
20.6 The Benefits of Active Process Migration
20.7 Pareto Distributions are Everywhere
20.8 Exercises
21 Phase-Type Workloads and Matrix-Analytic Methods
21.1 Representing General Distributions by Exponentials
21.2 Markov Chain Modeling of PH Workloads
21.3 The Matrix-Analytic method
21.4 Analysis of Time-Varying Load
21.4.1 High-Level Ideas
21.4.2 The Generator Matrix, Q
21.4.3 Solving for R
21.4.4 Finding Initial State Probability
21.4.5 Performance Metrics
21.5 More Complex Chains
21.6 Readings and Further Remarks
21.7 Exercises
22 Networks with Time-Sharing (PS) Servers (BCMP)
22.1 Review of Product-Form Networks
22.2 BCMP Result
22.2.1 Networks with FCFS Servers
22.2.2 Networks with PS Servers
22.3 M/M/1/PS
22.4 M/Cox/1/PS
22.5 Tandem Network of M/G/1/PS Servers
22.6 Network of PS Servers with Probabilistic Routing
22.7 Readings
22.8 Exercises
23 The M/G/1 Queue and the Inspection Paradox
23.1 The Inspection Paradox
23.2 The M/G/1 Queue and Its Analysis
23.3 Renewal-Reward Theory
23.4 Applying Renewal-Reward to Get Expected Excess
23.5 Back to the Inspection Paradox
23.6 Back to the M/G/1 Queue
23.7 Exercises
24 Task Assignment Policies for Server Farms
24.1 Task Assignment for FCFS Server Farms
24.2 Task Assignment for PS Server Farms
24.3 Optimal Server Farm Design
24.4 Readings and Further Followup
24.5 Exercises
25 Transform Analysis
25.1 Definitions of Transforms and Some Examples
25.2 Getting Moments from Transforms: Peeling the Onion
25.3 Linearity of Transforms
25.4 Conditioning
25.5 Distribution of Response Time In an M/M/1
25.6 Combining Laplace and z-Transforms
25.7 More Results on Transforms
25.8 Readings
25.9 Exercises
26 M/G/1 Transform Analysis
26.1 The z-Transform of the Number in System
26.2 The Laplace Transform of Time in System
26.3 Readings
26.4 Exercises
27 Power Optimization Application
27.1 The Power Optimization Problem
27.2 Busy Period Analysis of M/G/1
27.3 M/G/1 with Setup Cost
27.4 Comparing ON/IDLE versus ON/OFF
27.5 Readings
27.6 Exercises
Part VII: Smart Scheduling in the M/G/1
28 Performance Metrics
28.1 Traditional Metrics
28.2 Commonly Used Metrics for Single Queues
28.3 Todayās Trendy Metrics
28.4 Starvation/Fairness Metrics
28.5 Deriving Performance Metrics
28.6 Readings
29 Non-Preemptive, Non-Size-Based Policies
29.1 FCFS, LCFS, and RANDOM
29.2 Readings
29.3 Exercises
30 Preemptive, Non-Size-Based Policies
30.1 Processor-Sharing (PS)
30.1.1 Motivation behind PS
30.1.2 Ages of Jobs in the M/G/1/PS System
30.1.3 Response Time as a Function of Job Size
30.1.4 Intuition for PS Results
30.1.5 Implications of PS Result on Understanding FCFS
30.2 Preemptive-LCFS
30.3 FB Scheduling
30.4 Readings
30.5 Exercises
31 Non-Preemptive, Size-Based Policies
31.1 Priority Queueing
31.2 Non-Preemptive Priority
31.3 Shortest Job First (SJF)
31.4 The Problem with Non-Preemptive Policies
31.5 Exercises
32 Preemptive, Size-Based Policies
32.1 Motivation
32.2 Preemptive Priority Queueing
32.3 Preemptive-Shortest-Job-First (PSJF)
32.4 Transform Analysis of PSJF
32.5 Exercises
33 Scheduling: SRPT and Fairness
33.1 Shortest Remaining Processing Time (SRPT)
33.2 Precise Derivation of SRPT Waiting Time
33.3 Comparisons with Other Policies
33.3.1 Comparison with PSJF
33.3.2 SRPT versus FB
33.3.3 Comparison of All Scheduling Policies
33.4 Fairness of SRPT
33.5 Readings
Part I: Introduction to Queueing
1 Motivating Examples of the Power of Analytical Modeling
1.1 What is Queueing Theory?
1.2 Examples of the Power of Queueing Theory
2 Queueing Theory Terminology
2.1 Where We Are Heading
2.2 The Single-Server Network
2.3 Classification of Queueing Networks
2.4 Open Networks
2.5 More Metrics: Throughput and Utilization
2.6 Closed Networks
2.6.1 Interactive (Terminal-Driven) System
2.6.2 Batch Systems
2.6.3 Throughput in a Closed System
2.7 Differences between Closed and Open Networks
2.7.1 A Question on Modeling
2.8 Related Readings
2.9 Exercises
Part II: Necessary Probability Background
3 Probability Review
3.1 Sample Space and Events
3.2 Probability Defined on Events
3.3 Conditional Probabilities on Events
3.4 Independent Events and Conditionally Independent Events
3.5 Law of Total Probability
3.6 Bayes Law
3.7 Discrete versus Continuous Random Variables
3.8 Probabilities and Densities
3.8.1 Discrete: Probability Mass Function
3.8.2 Continuous: Probability Density Function
3.9 Expectation and Variance
3.10 Joint Probabilities and Independence
3.11 Conditional Probabilities and Expectations
3.12 Probabilities and Expectations via Conditioning
3.13 Linearity of Expectation
3.14 Normal Distribution
3.14.1 Linear Transformation Property
3.14.2 Central Limit Theorem
3.15 Sum of a Random Number of Random Variables
3.16 Exercises
4 Generating Random Variables
4.1 Inverse Transform Method
4.1.1 The Continuous Case
4.1.2 The Discrete Case
4.2 Accept/Reject Method
4.2.1 Discrete Case
4.2.2 Continuous Case
4.2.3 Some Hard Problems
4.3 Readings
4.4 Exercises
5 Sample Paths, Convergence, and Averages
5.1 Convergence
5.2 Strong and Weak Laws of Large Numbers
5.3 Time Average versus Ensemble Average
5.3.1 Motivation
5.3.2 Definition
5.3.3 Interpretation
5.3.4 Equivalence
5.3.5 Simulation
5.3.6 Average Time in System
5.4 Related readings
5.5 Exercises
Part III: The Predictive Power of Simple Operational Laws: What-If Questions and Answers
6 Little's Law and Other Operational Laws
6.1 Little's Law for Open Systems
6.2 Intuitions
6.3 Little's Law for Closed Systems
6.4 Open Systems
6.4.1 Statement via Time Averages
6.4.2 Proof
6.4.3 Corollaries
6.5 Closed Systems
6.5.1 Statement via Time Averages
6.5.2 Proof
6.6 Generalized Littleās Law
6.7 Examples Applying Little's Law
6.8 More Operational Laws: The Forced Flow Law
6.9 Combining Operational Laws
6.10 Device Demands
6.11 Readings and Further Topics Related to Little's Law
6.12 Exercises
7 Modification Analysis: What-if for Closed Systems
7.1 Review
7.2 Asymptotic Bounds for Closed Systems
7.3 Modification Analysis for Closed Systems
7.4 More Modification Analysis Examples
7.5 Comparison of Closed and Open Networks
7.6 Readings
7.7 Exercises
Part IV: From Markov Chains to Simple Queues
8 Discrete-Time Markov Chains
8.1 Discrete-Time versus Continuous-Time Markov Chains
8.2 Definition of a DTMC
8.3 Examples of Finite-State DTMCs
8.3.1 Repair Facility Problem
8.3.2 Umbrella Problem
8.3.3 Program Analysis Problem
8.4 Powers of P: n-Step Transition Probabilities
8.5 Stationary Equations
8.6 The Stationary Distribution Equals the Limiting Distribution
8.7 Examples of Solving Stationary Equations
8.7.1 Repair Facility Problem with Cost
8.7.2 Umbrella Problem
8.8 Infinite-State DTMCs
8.9 Infinite-State Stationarity Result
8.10 Solving Stationary Equations in Infinite-State DTMCs
8.11 Exercises
9 Ergodicity Theory
9.1 Ergodicity Questions
9.2 Finite-State DTMCs
9.2.1 Existence of the Limiting Distribution
9.2.2 Mean Time between Visits to a State
9.2.3 Time Averages
9.3 Infinite-State Markov Chains
9.3.1 Recurrent versus Transient
9.3.2 Infinite Random Walk Example
9.3.3 Positive Recurrent versus Null Recurrent
9.3.4 Relating Limiting Probabilities to Stationary Probabilities
9.4 Ergodic Theorem of Markov Chains
9.5 Time Averages
9.6 Limiting Probabilities Interpreted as Rates
9.7 Time-Reversibility Theorem
9.8 When Chains are Periodic or not Irreducible
9.8.1 Periodic Chains
9.8.2 Chains that are not Irreducible
9.9 Conclusion
9.10 Proof of Ergodic Theorem of Markov Chains
9.11 Exercises
10 Real-World Examples: Google, Aloha, and Harder Chains
10.1 Google's PageRank algorithm
10.1.1 Google's DTMC Algorithm
10.1.2 Problems with Real Web Graphs
10.1.3 Googleās Solution to Dead Ends and Spider Traps
10.1.4 Evaluation of the PageRank Algorithm
10.1.5 Practical Implementation Considerations
10.2 Aloha Protocol Analysis
10.2.1 The Slotted Aloha Protocol
10.2.2 The Aloha Markov Chain
10.2.3 Properties of the Aloha Markov Chain
10.2.4 Improving the Aloha Protocol
10.3 Generating Functions for Harder Markov Chains
10.3.1 The z-Transform
10.3.2 Solving the Chain
10.4 Readings and Summary
10.5 Exercises
11 Exponential Distribution and the Poisson Process
11.1 Definition of the Exponential Distribution
11.2 Memoryless Property of the Exponential
11.3 Relating Exponential to Geometric
11.4 More Properties of the Exponential
11.5 The Celebrated Poisson Process
11.6 Merging Independent Poisson Processes
11.7 Poisson Splitting
11.8 Uniformity
11.9 Exercises
12 Transition to Continuous-Time Markov Chains
12.1 Defining CTMCs
12.2 Solving CTMCs
12.3 Generalization and Interpretation
12.3.1 Interpreting the Balance Equations for the CTMC
12.3.2 Summary Theorem for CTMCs
12.4 Exercises
13 M/M/1 and PASTA
13.1 The M/M/1 Queue
13.2 Examples Using an M/M/1 Queue
13.3 PASTA
13.4 Further Reading
13.5 Exercises
Part V: Server Farms and Networks: Multi-Server, Multi-Queue Systems
14 Server Farms: M/M/k and M/M/k/k
14.1 Time-Reversibility for CTMCs
14.2 M/M/k/k Loss System
14.3 M/M/k
14.4 Comparison of Three Server Organizations
14.5 Readings
14.6 Exercises
15 Capacity Provisioning for Server Farms
15.1 What Does Load Really Mean in an M/M/k?
15.2 The M/M/1
15.2.1 Analysis of the M/M/1
15.2.2 A First Cut at a Capacity Provisioning Rule for the M/M/k
15.3 Square-Root Staffing
15.4 Readings
15.5 Exercises
16 Time-Reversibility and Burke's Theorem
16.1 More Examples of Finite-State CTMCs
16.1.1 Networks with Finite Buffer Space
16.1.2 Batch System with M/M/2 I/O
16.2 The Reverse Chain
16.3 Burkeās Theorem
16.4 An Alternative (Partial) Proof of Burke's Theorem
16.5 Application: Tandem Servers
16.6 General Acyclic Networks with Probabilistic Routing
16.7 Readings
16.8 Exercises
17 Networks of Queues and Jackson Product Form
17.1 Jackson Network Definition
17.2 The Arrival Process into Each Server
17.3 Solving the Jackson Network
17.4 The Local Balance Approach
17.5 Readings
17.6 Exercises
18 Classed Network of Queues
18.1 Overview
18.2 Motivation for Classed Networks
18.3 Notation and Modeling for Classed Jackson Networks
18.4 A Single-Server Classed Network
18.5 Product Form Theorems
18.6 Examples Using Classed Networks
18.6.1 Connection-Oriented Network Example
18.6.2 Distribution of Job Classes Example
18.6.3 CPU-Bound and I/O-Bound Jobs Example
18.7 Readings
18.8 Exercises
19 Closed Networks of Queues
19.1 Motivation
19.2 Product-Form Solution
19.2.1 Local Balance Equations for Closed Networks
19.2.2 Example of Deriving Limiting Probabilities
19.3 Mean Value Analysis (MVA)
19.3.1 The Arrival Theorem:
19.3.2 Iterative Derivation of Mean Response Time
19.3.3 An MVA Example
19.4 Readings
19.5 Exercises
Part VI: Real-WorldWorkloads: High Variability and Heavy Tails
20 Tales of Tails: Real-World Workloads
20.1 Grad School Tales ... Process Migration
20.2 UNIX Process Lifetime Measurements
20.3 Properties of the Pareto Distribution
20.4 The Bounded Pareto distribution
20.5 Heavy Tails
20.6 The Benefits of Active Process Migration
20.7 Pareto Distributions are Everywhere
20.8 Exercises
21 Phase-Type Workloads and Matrix-Analytic Methods
21.1 Representing General Distributions by Exponentials
21.2 Markov Chain Modeling of PH Workloads
21.3 The Matrix-Analytic method
21.4 Analysis of Time-Varying Load
21.4.1 High-Level Ideas
21.4.2 The Generator Matrix, Q
21.4.3 Solving for R
21.4.4 Finding Initial State Probability
21.4.5 Performance Metrics
21.5 More Complex Chains
21.6 Readings and Further Remarks
21.7 Exercises
22 Networks with Time-Sharing (PS) Servers (BCMP)
22.1 Review of Product-Form Networks
22.2 BCMP Result
22.2.1 Networks with FCFS Servers
22.2.2 Networks with PS Servers
22.3 M/M/1/PS
22.4 M/Cox/1/PS
22.5 Tandem Network of M/G/1/PS Servers
22.6 Network of PS Servers with Probabilistic Routing
22.7 Readings
22.8 Exercises
23 The M/G/1 Queue and the Inspection Paradox
23.1 The Inspection Paradox
23.2 The M/G/1 Queue and Its Analysis
23.3 Renewal-Reward Theory
23.4 Applying Renewal-Reward to Get Expected Excess
23.5 Back to the Inspection Paradox
23.6 Back to the M/G/1 Queue
23.7 Exercises
24 Task Assignment Policies for Server Farms
24.1 Task Assignment for FCFS Server Farms
24.2 Task Assignment for PS Server Farms
24.3 Optimal Server Farm Design
24.4 Readings and Further Followup
24.5 Exercises
25 Transform Analysis
25.1 Definitions of Transforms and Some Examples
25.2 Getting Moments from Transforms: Peeling the Onion
25.3 Linearity of Transforms
25.4 Conditioning
25.5 Distribution of Response Time In an M/M/1
25.6 Combining Laplace and z-Transforms
25.7 More Results on Transforms
25.8 Readings
25.9 Exercises
26 M/G/1 Transform Analysis
26.1 The z-Transform of the Number in System
26.2 The Laplace Transform of Time in System
26.3 Readings
26.4 Exercises
27 Power Optimization Application
27.1 The Power Optimization Problem
27.2 Busy Period Analysis of M/G/1
27.3 M/G/1 with Setup Cost
27.4 Comparing ON/IDLE versus ON/OFF
27.5 Readings
27.6 Exercises
Part VII: Smart Scheduling in the M/G/1
28 Performance Metrics
28.1 Traditional Metrics
28.2 Commonly Used Metrics for Single Queues
28.3 Todayās Trendy Metrics
28.4 Starvation/Fairness Metrics
28.5 Deriving Performance Metrics
28.6 Readings
29 Non-Preemptive, Non-Size-Based Policies
29.1 FCFS, LCFS, and RANDOM
29.2 Readings
29.3 Exercises
30 Preemptive, Non-Size-Based Policies
30.1 Processor-Sharing (PS)
30.1.1 Motivation behind PS
30.1.2 Ages of Jobs in the M/G/1/PS System
30.1.3 Response Time as a Function of Job Size
30.1.4 Intuition for PS Results
30.1.5 Implications of PS Result on Understanding FCFS
30.2 Preemptive-LCFS
30.3 FB Scheduling
30.4 Readings
30.5 Exercises
31 Non-Preemptive, Size-Based Policies
31.1 Priority Queueing
31.2 Non-Preemptive Priority
31.3 Shortest Job First (SJF)
31.4 The Problem with Non-Preemptive Policies
31.5 Exercises
32 Preemptive, Size-Based Policies
32.1 Motivation
32.2 Preemptive Priority Queueing
32.3 Preemptive-Shortest-Job-First (PSJF)
32.4 Transform Analysis of PSJF
32.5 Exercises
33 Scheduling: SRPT and Fairness
33.1 Shortest Remaining Processing Time (SRPT)
33.2 Precise Derivation of SRPT Waiting Time
33.3 Comparisons with Other Policies
33.3.1 Comparison with PSJF
33.3.2 SRPT versus FB
33.3.3 Comparison of All Scheduling Policies
33.4 Fairness of SRPT
33.5 Readings