注重体验与质量的电子书资源下载网站
分类于: 职场办公 设计
简介
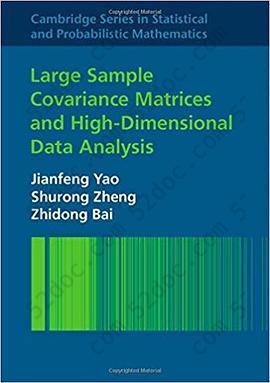
Large Sample Covariance Matrices and High-Dimensional Data Analysis 豆 0.0分
资源最后更新于 2020-09-05 22:00:22
作者:Jianfeng Yao
出版社:Cambridge University Press
出版日期:2015-01
ISBN:9781107065178
文件格式: pdf
标签: 统计学习 Statistical 2016
简介· · · · · ·
Book description
High-dimensional data appear in many fields, and their analysis has become increasingly important in modern statistics. However, it has long been observed that several well-known methods in multivariate analysis become inefficient, or even misleading, when the data dimension p is larger than, say, several tens. A seminal example is the well-known inefficiency o...
目录
http://web.hku.hk/~jeffyao/docs/samplechaps-scv-Aug23.pdf
Notations page vi
Preface vii
1 Introduction 1
1.1 Large dimensional data and new asymptotic statistics 1
1.2 Random matrix theory 3
1.3 Eigenvalue statistics of large sample covariance matrices 4
1.4 Organisation of the book 5
2 Limiting spectral distributions 7
2.1 Introduction 7
2.2 Fundamental tools 8
2.3 Marcenko-Pastur distributions ˇ 10
2.4 Generalised Marcenko-Pastur distributions 16 ˇ
2.5 LSD for random Fisher matrices 22
3 CLT for linear spectral statistics 30
3.1 Introduction 30
3.2 CLT for linear spectral statistics of a sample covariance matrix 31
3.3 Bai and Silverstein’s CLT 39
3.4 CLT for linear spectral statistics of random Fisher matrices 40
3.5 The substitution principle 44
4 The generalised variance and multiple correlation coefficient 47
4.1 Introduction 47
4.2 The generalised variance 47
4.3 The multiple correlation coefficient 52
5 The T-statistic 57
5.1 Introduction 57
5.2 Dempster’s non-exact test 58
5.3 Bai-Saranadasa’s test 60
5.4 Improvements of the Bai-Saranadasa test 62
5.5 Monte-Carlo results 66
6 Classification of data 69
6.1 Introduction 69
6.2 Classification into one of two known multivariate normal populations 69
6.3 Classification into one of two multivariate normal populations
with unknown parameters 70
6.4 Classification into one of several multivariate normal populations 72
6.5 Classification under large dimensions: the T-rule and the D-rule 73
6.6 Misclassification rate of the D-rule in case of two normal populations 74
6.7 Misclassification rate of the T-rule in case of two normal populations 77
6.8 Comparison between the T-rule and the D-rule 78
6.9 Misclassification rate of the T-rule in case of two general populations 79
6.10 Misclassification rate of the D-rule in case of two general populations 83
6.11 Simulation study 89
6.12 A real data analysis 94
7 Testing the general linear hypothesis 97
7.1 Introduction 97
7.2 Estimators of parameters in multivariate linear regression 98
7.3 Likelihood ratio criteria for testing linear hypotheses about
regression coefficients 98
7.4 The distribution of the likelihood ratio criterion under the null 99
7.5 Testing equality of means of several normal distributions with
common covariance matrix 101
7.6 Large regression analysis 103
7.7 A large-dimensional multiple sample significance test 109
8 Testing independence of sets of variates 115
8.1 Introduction 115
8.2 The likelihood ratio criterion 115
8.3 The distribution of the likelihood ratio criterion under the null
hypothesis 118
8.4 The case of two sets of variates 120
8.5 Testing independence of two sets of many variates 122
8.6 Testing independence of more than two sets of many variates 126
9 Testing hypotheses of equality of covariance matrices 130
9.1 Introduction 130
9.2 Criteria for testing equality of several covariance matrices 130
9.3 Criteria for testing that several normal distributions are identical 133
9.4 The sphericity test 136
9.5 Testing the hypothesis that a covariance matrix is equal to a given
matrix 138
9.6 Testing hypotheses of equality of large-dimensional covariance
matrices 139
9.7 Large-dimensional sphericity test 148
10 Estimation of the population spectral distribution 160
10.1 Introduction 160
10.2 A method-of-moments estimator 161
10.3 An estimator using least sum of squares 166
10.4 A local moment estimator 176
10.5 A cross-validation method for selection of the order of a population
spectral distribution 189
11 Large-dimensional spiked population models 201
11.1 Introduction 201
11.2 Limits of spiked sample eigenvalues 203
11.3 Limits of spiked sample eigenvectors 209
11.4 Central limit theorem for spiked sample eigenvalues 211
11.5 Estimation of the values of spike eigenvalues 224
11.6 Estimation of the number of spike eigenvalues 226
11.7 Estimation of the noise variance 237
12 Efficient optimisation of a large financial portfolio 244
12.1 Introduction 244
12.2 Mean-Variance Principle and the Markowitz’s enigma 244
12.3 The plug-in portfolio and over-prediction of return 247
12.4 Bootstrap enhancement to the plug-in portfolio 253
12.5 Spectrum-corrected estimators 257
Appendix A Curvilinear integrals 275
Appendix B Eigenvalue inequalities 282
Bibliography 285
Index 291
Notations page vi
Preface vii
1 Introduction 1
1.1 Large dimensional data and new asymptotic statistics 1
1.2 Random matrix theory 3
1.3 Eigenvalue statistics of large sample covariance matrices 4
1.4 Organisation of the book 5
2 Limiting spectral distributions 7
2.1 Introduction 7
2.2 Fundamental tools 8
2.3 Marcenko-Pastur distributions ˇ 10
2.4 Generalised Marcenko-Pastur distributions 16 ˇ
2.5 LSD for random Fisher matrices 22
3 CLT for linear spectral statistics 30
3.1 Introduction 30
3.2 CLT for linear spectral statistics of a sample covariance matrix 31
3.3 Bai and Silverstein’s CLT 39
3.4 CLT for linear spectral statistics of random Fisher matrices 40
3.5 The substitution principle 44
4 The generalised variance and multiple correlation coefficient 47
4.1 Introduction 47
4.2 The generalised variance 47
4.3 The multiple correlation coefficient 52
5 The T-statistic 57
5.1 Introduction 57
5.2 Dempster’s non-exact test 58
5.3 Bai-Saranadasa’s test 60
5.4 Improvements of the Bai-Saranadasa test 62
5.5 Monte-Carlo results 66
6 Classification of data 69
6.1 Introduction 69
6.2 Classification into one of two known multivariate normal populations 69
6.3 Classification into one of two multivariate normal populations
with unknown parameters 70
6.4 Classification into one of several multivariate normal populations 72
6.5 Classification under large dimensions: the T-rule and the D-rule 73
6.6 Misclassification rate of the D-rule in case of two normal populations 74
6.7 Misclassification rate of the T-rule in case of two normal populations 77
6.8 Comparison between the T-rule and the D-rule 78
6.9 Misclassification rate of the T-rule in case of two general populations 79
6.10 Misclassification rate of the D-rule in case of two general populations 83
6.11 Simulation study 89
6.12 A real data analysis 94
7 Testing the general linear hypothesis 97
7.1 Introduction 97
7.2 Estimators of parameters in multivariate linear regression 98
7.3 Likelihood ratio criteria for testing linear hypotheses about
regression coefficients 98
7.4 The distribution of the likelihood ratio criterion under the null 99
7.5 Testing equality of means of several normal distributions with
common covariance matrix 101
7.6 Large regression analysis 103
7.7 A large-dimensional multiple sample significance test 109
8 Testing independence of sets of variates 115
8.1 Introduction 115
8.2 The likelihood ratio criterion 115
8.3 The distribution of the likelihood ratio criterion under the null
hypothesis 118
8.4 The case of two sets of variates 120
8.5 Testing independence of two sets of many variates 122
8.6 Testing independence of more than two sets of many variates 126
9 Testing hypotheses of equality of covariance matrices 130
9.1 Introduction 130
9.2 Criteria for testing equality of several covariance matrices 130
9.3 Criteria for testing that several normal distributions are identical 133
9.4 The sphericity test 136
9.5 Testing the hypothesis that a covariance matrix is equal to a given
matrix 138
9.6 Testing hypotheses of equality of large-dimensional covariance
matrices 139
9.7 Large-dimensional sphericity test 148
10 Estimation of the population spectral distribution 160
10.1 Introduction 160
10.2 A method-of-moments estimator 161
10.3 An estimator using least sum of squares 166
10.4 A local moment estimator 176
10.5 A cross-validation method for selection of the order of a population
spectral distribution 189
11 Large-dimensional spiked population models 201
11.1 Introduction 201
11.2 Limits of spiked sample eigenvalues 203
11.3 Limits of spiked sample eigenvectors 209
11.4 Central limit theorem for spiked sample eigenvalues 211
11.5 Estimation of the values of spike eigenvalues 224
11.6 Estimation of the number of spike eigenvalues 226
11.7 Estimation of the noise variance 237
12 Efficient optimisation of a large financial portfolio 244
12.1 Introduction 244
12.2 Mean-Variance Principle and the Markowitz’s enigma 244
12.3 The plug-in portfolio and over-prediction of return 247
12.4 Bootstrap enhancement to the plug-in portfolio 253
12.5 Spectrum-corrected estimators 257
Appendix A Curvilinear integrals 275
Appendix B Eigenvalue inequalities 282
Bibliography 285
Index 291