注重体验与质量的电子书资源下载网站
分类于: 职场办公 计算机基础
简介
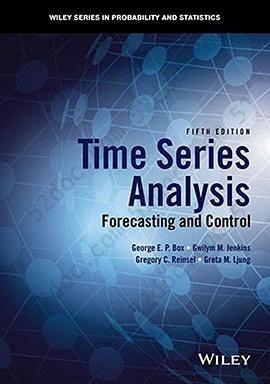
Time Series Analysis: Forecasting and Control 豆 0.0分
资源最后更新于 2020-09-05 22:03:22
作者:George E. P. Box
出版社:Wiley
出版日期:2008-01
ISBN:9781118675021
文件格式: pdf
标签: 金融数学 数学 Mathematics 英文原版 统计学 时间序列 教材 textbook統計
简介· · · · · ·
A modernized new edition of one of the most trusted books on time series analysis. Since publication of the first edition in 1970, Time Series Analysis has served as one of the most influential and prominent works on the subject. This new edition maintains its balanced presentation of the tools for modeling and analyzing time series and also introduces the latest developments t...
目录
Table of Contents
Preface to the Fourth Edition xxi
Preface to the Third Edition xxiii
1 Introduction 1
1.1 Five Important Practical Problems, 2
1.2 Stochastic and Deterministic Dynamic Mathematical Models, 7
1.3 Basic Ideas in Model Building, 16
Part One Stochastic Models and Their Forecasting 19
2 Autocorrelation Function and Spectrum of Stationary Processes 21
2.1 Autocorrelation Properties of Stationary Models, 21
2.2 Spectral Properties of Stationary Models, 35
3 Linear Stationary Models 47
3.1 General Linear Process, 47
3.2 Autoregressive Processes, 55
3.3 Moving Average Processes, 71
3.4 Mixed Autoregressive–Moving Average Processes, 79
4 Linear Nonstationary Models 93
4.1 Autoregressive Integrated Moving Average Processes, 93
4.2 Three Explicit Forms for The Autoregressive Integrated Moving Average Model, 103
4.3 Integrated Moving Average Processes, 114
5 Forecasting 137
5.1 Minimum Mean Square Error Forecasts and Their Properties, 137
5.2 Calculating and Updating Forecasts, 145
5.3 Forecast Function and Forecast Weights, 152
5.4 Examples of Forecast Functions and Their Updating, 157
5.5 Use of State-Space Model Formulation for Exact Forecasting, 170
5.6 Summary, 177
Part Two Stochastic Model Building 193
6 Model Identification 195
6.1 Objectives of Identification, 195
6.2 Identification Techniques, 196
6.3 Initial Estimates for the Parameters, 213
6.4 Model Multiplicity, 221
7 Model Estimation 231
7.1 Study of the Likelihood and Sum-of-Squares Functions, 231
7.2 Nonlinear Estimation, 255
7.3 Some Estimation Results for Specific Models, 268
7.4 Likelihood Function Based on the State-Space Model, 275
7.5 Unit Roots in Arima Models, 280
7.6 Estimation Using Bayes’s Theorem, 287
8 Model Diagnostic Checking 333
8.1 Checking the Stochastic Model, 333
8.2 Diagnostic Checks Applied to Residuals, 335
8.3 Use of Residuals to Modify the Model, 350
9 Seasonal Models 353
9.1 Parsimonious Models for Seasonal Time Series, 353
9.2 Representation of the Airline Data by a Multiplicative (0, 1, 1) × (0, 1, 1)12 Model, 359
9.3 Some Aspects of More General Seasonal ARIMA Models, 375
9.4 Structural Component Models and Deterministic Seasonal Components, 384
9.5 Regression Models with Time Series Error Terms, 397
10 Nonlinear and Long Memory Models 413
10.1 Autoregressive Conditional Heteroscedastic (ARCH) Models, 413
10.2 Nonlinear Time Series Models, 420
10.3 Long Memory Time Series Processes, 428
Part Three Transfer Function and Multivariate Model Building 437
11 Transfer Function Models 439
11.1 Linear Transfer Function Models, 439
11.2 Discrete Dynamic Models Represented by Difference Equations, 447
11.3 Relation Between Discrete and Continuous Models, 458
12 Identification, Fitting, and Checking of Transfer Function Models 473
12.1 Cross-Correlation Function, 474
12.2 Identification of Transfer Function Models, 481
12.3 Fitting and Checking Transfer Function Models, 492
12.4 Some Examples of Fitting and Checking Transfer Function Models, 501
12.5 Forecasting With Transfer Function Models Using Leading Indicators, 509
12.6 Some Aspects of the Design of Experiments to Estimate Transfer Functions, 519
13 Intervention Analysis Models and Outlier Detection 529
13.1 Intervention Analysis Methods, 529
13.2 Outlier Analysis for Time Series, 536
13.3 Estimation for ARMA Models with Missing Values, 543
14 Multivariate Time Series Analysis 551
14.1 Stationary Multivariate Time Series, 552
14.2 Linear Model Representations for Stationary Multivariate Processes, 556
14.3 Nonstationary Vector Autoregressive–Moving Average Models, 570
14.4 Forecasting for Vector Autoregressive–Moving Average Processes, 573
14.5 State-Space Form of the Vector ARMA Model, 575
14.6 Statistical Analysis of Vector ARMA Models, 578
14.7 Example of Vector ARMA Modeling, 588
Part Four Design of Discrete Control Schemes 597
15 Aspects of Process Control 599
15.1 Process Monitoring and Process Adjustment, 600
15.2 Process Adjustment Using Feedback Control, 604
15.3 Excessive Adjustment Sometimes Required by MMSE Control, 620
15.4 Minimum Cost Control with Fixed Costs of Adjustment and Monitoring, 623
15.5 Feedforward Control, 627
15.6 Monitoring Values of Parameters of Forecasting and Feedback Adjustment Schemes, 642
Part Five Charts and Tables 659
Collection of Tables and Charts 661
Collection of Time Series Used for Examples in the Text and in Exercises 669
References 685
Part Six Exercises and Problems 701
Index 729
Preface to the Fourth Edition xxi
Preface to the Third Edition xxiii
1 Introduction 1
1.1 Five Important Practical Problems, 2
1.2 Stochastic and Deterministic Dynamic Mathematical Models, 7
1.3 Basic Ideas in Model Building, 16
Part One Stochastic Models and Their Forecasting 19
2 Autocorrelation Function and Spectrum of Stationary Processes 21
2.1 Autocorrelation Properties of Stationary Models, 21
2.2 Spectral Properties of Stationary Models, 35
3 Linear Stationary Models 47
3.1 General Linear Process, 47
3.2 Autoregressive Processes, 55
3.3 Moving Average Processes, 71
3.4 Mixed Autoregressive–Moving Average Processes, 79
4 Linear Nonstationary Models 93
4.1 Autoregressive Integrated Moving Average Processes, 93
4.2 Three Explicit Forms for The Autoregressive Integrated Moving Average Model, 103
4.3 Integrated Moving Average Processes, 114
5 Forecasting 137
5.1 Minimum Mean Square Error Forecasts and Their Properties, 137
5.2 Calculating and Updating Forecasts, 145
5.3 Forecast Function and Forecast Weights, 152
5.4 Examples of Forecast Functions and Their Updating, 157
5.5 Use of State-Space Model Formulation for Exact Forecasting, 170
5.6 Summary, 177
Part Two Stochastic Model Building 193
6 Model Identification 195
6.1 Objectives of Identification, 195
6.2 Identification Techniques, 196
6.3 Initial Estimates for the Parameters, 213
6.4 Model Multiplicity, 221
7 Model Estimation 231
7.1 Study of the Likelihood and Sum-of-Squares Functions, 231
7.2 Nonlinear Estimation, 255
7.3 Some Estimation Results for Specific Models, 268
7.4 Likelihood Function Based on the State-Space Model, 275
7.5 Unit Roots in Arima Models, 280
7.6 Estimation Using Bayes’s Theorem, 287
8 Model Diagnostic Checking 333
8.1 Checking the Stochastic Model, 333
8.2 Diagnostic Checks Applied to Residuals, 335
8.3 Use of Residuals to Modify the Model, 350
9 Seasonal Models 353
9.1 Parsimonious Models for Seasonal Time Series, 353
9.2 Representation of the Airline Data by a Multiplicative (0, 1, 1) × (0, 1, 1)12 Model, 359
9.3 Some Aspects of More General Seasonal ARIMA Models, 375
9.4 Structural Component Models and Deterministic Seasonal Components, 384
9.5 Regression Models with Time Series Error Terms, 397
10 Nonlinear and Long Memory Models 413
10.1 Autoregressive Conditional Heteroscedastic (ARCH) Models, 413
10.2 Nonlinear Time Series Models, 420
10.3 Long Memory Time Series Processes, 428
Part Three Transfer Function and Multivariate Model Building 437
11 Transfer Function Models 439
11.1 Linear Transfer Function Models, 439
11.2 Discrete Dynamic Models Represented by Difference Equations, 447
11.3 Relation Between Discrete and Continuous Models, 458
12 Identification, Fitting, and Checking of Transfer Function Models 473
12.1 Cross-Correlation Function, 474
12.2 Identification of Transfer Function Models, 481
12.3 Fitting and Checking Transfer Function Models, 492
12.4 Some Examples of Fitting and Checking Transfer Function Models, 501
12.5 Forecasting With Transfer Function Models Using Leading Indicators, 509
12.6 Some Aspects of the Design of Experiments to Estimate Transfer Functions, 519
13 Intervention Analysis Models and Outlier Detection 529
13.1 Intervention Analysis Methods, 529
13.2 Outlier Analysis for Time Series, 536
13.3 Estimation for ARMA Models with Missing Values, 543
14 Multivariate Time Series Analysis 551
14.1 Stationary Multivariate Time Series, 552
14.2 Linear Model Representations for Stationary Multivariate Processes, 556
14.3 Nonstationary Vector Autoregressive–Moving Average Models, 570
14.4 Forecasting for Vector Autoregressive–Moving Average Processes, 573
14.5 State-Space Form of the Vector ARMA Model, 575
14.6 Statistical Analysis of Vector ARMA Models, 578
14.7 Example of Vector ARMA Modeling, 588
Part Four Design of Discrete Control Schemes 597
15 Aspects of Process Control 599
15.1 Process Monitoring and Process Adjustment, 600
15.2 Process Adjustment Using Feedback Control, 604
15.3 Excessive Adjustment Sometimes Required by MMSE Control, 620
15.4 Minimum Cost Control with Fixed Costs of Adjustment and Monitoring, 623
15.5 Feedforward Control, 627
15.6 Monitoring Values of Parameters of Forecasting and Feedback Adjustment Schemes, 642
Part Five Charts and Tables 659
Collection of Tables and Charts 661
Collection of Time Series Used for Examples in the Text and in Exercises 669
References 685
Part Six Exercises and Problems 701
Index 729