注重体验与质量的电子书资源下载网站
分类于: 其它 计算机基础
简介
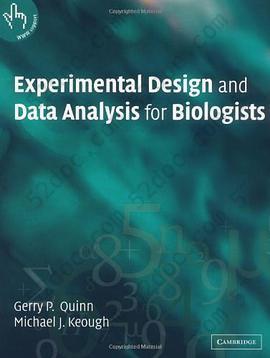
Experimental Design and Data Analysis for Biologists 豆 0.0分
资源最后更新于 2020-10-05 18:40:08
作者:Quinn, G. P./ Keough, Michael J.
出版社:Cambridge University Press
出版日期:2002-01
ISBN:9780521009768
文件格式: pdf
标签: 专业书书单 生物 未完成 数据科学 S_统计学习 Biostat
简介· · · · · ·
An essential textbook for any student or researcher in biology needing to design experiments, sample programs or analyse the resulting data. The text begins with a revision of estimation and hypothesis testing methods, covering both classical and Bayesian philosophies, before advancing to the analysis of linear and generalized linear models. Topics covered include linear and lo...
目录
1 Introduction
1.1 Scientific method
1.2 Experiments and other tests
1.3 Data, observations and variables
1.4 Probability
1.5 Probability distributions
2 Estimation
2.1 Samples and populations
2.2 Common parameters and statistics
2.3 Standard errors and confidence intervals for the mean
2.4 Methods for estimating parameters
2.5 Resampling methods for estimation
2.6 Bayesian inference – estimation
3 Hypothesis testing
3.1 Statistical hypothesis testing
3.2 Decision errors
3.3 Other testing methods
3.4 Multiple testing
3.5 Combining results from statistical tests
3.6 Critique of statistical hypothesis testing
3.7 Bayesian hypothesis testing
4 Graphical exploration of data
4.1 Exploratory data analysis
4.2 Analysis with graphs
4.3 Transforming data
4.4 Standardizations
4.5 Outliers
4.6 Censored and missing data
4.7 General issues and hints for analysis
5 Correlation and regression
5.1 Correlation analysis
5.2 Linear models
5.3 Linear regression analysis
5.4 Relationship between regression and correlation
5.5 Smoothing
5.6 Power of tests in correlation and regression
5.7 General issues and hints for analysis
6 Multiple and complex regression
6.1 Multiple linear regression analysis
6.2 Regression trees
6.3 Path analysis and structural equation modeling
6.4 Nonlinear models
6.5 Smoothing and response surfaces
6.6 General issues and hints for analysis
7 Design and power analysis
7.1 Sampling
7.2 Experimental design
7.3 Power analysis
7.4 General issues and hints for analysis
8 Comparing groups or treatments – analysis of variance
8.1 Single factor (one way) designs
8.2 Factor effects
8.3 Assumptions
8.4 ANOVA diagnostics
8.5 Robust ANOVA
8.6 Specific comparisons of means
8.7 Tests for trends
8.8 Testing equality of group variances
8.9 Power of single factor ANOVA
8.10 General issues and hints for analysis
9 Multifactor analysis of variance
9.1 Nested (hierarchical) designs
9.2 Factorial designs
9.3 Pooling in multifactor designs
9.4 Relationship between factorial and nested designs
9.5 General issues and hints for analysis
10 Randomized blocks and simple repeated measures: unreplicated two factor designs
10.1 Unreplicated two factor experimental designs
10.2 Analyzing RCB and RM designs
10.3 Interactions in RCB and RM models
10.4 Assumptions
10.5 Robust RCB and RM analyses
10.6 Specific comparisons
10.7 Efficiency of blocking (to block or not to block?)
10.8 Time as a blocking factor
10.9 Analysis of unbalanced RCB designs
10.10 Power of RCB or simple RM designs
10.11 More complex block designs
10.12 Generalized randomized block designs
10.13 RCB and RM designs and statistical software
10.14 General issues and hints for analysis
11 Split-plot and repeated measures designs: partly nested analyses of variance
11.1 Partly nested designs
11.2 Analyzing partly nested designs
11.3 Assumptions
11.4 Robust partly nested analyses
11.5 Specific comparisons
11.6 Analysis of unbalanced partly nested designs
11.7 Power for partly nested designs
11.8 More complex designs
11.9 Partly nested designs and statistical software
11.10 General issues and hints for analysi
12 Analyses of covariance
12.1 Single factor analysis of covariance (ANCOVA)
12.2 Assumptions of ANCOVA
12.3 Homogeneous slopes
12.4 Robust ANCOVA
12.5 Unequal sample sizes (unbalanced designs)
12.6 Specific comparisons of adjusted means
12.7 More complex designs
12.8 General issues and hints for analysis
13 Generalized linear models and logistic regression
13.1 Generalized linear models
13.2 Logistic regression
13.3 Poisson regression
13.4 Generalized additive models
13.5 Models for correlated data
13.6 General issues and hints for analysis
14 Analyzing frequencies
14.1 Single variable goodness-of-fit tests
14.2 Contingency tables
14.3 Log-linear models
14.4 General issues and hints for analysis
15 Introduction to multivariate analyses
15.1 Multivariate data
15.2 Distributions and associations
15.3 Linear combinations, eigenvectors and eigenvalues
15.4 Multivariate distance and dissimilarity measures
15.5 Comparing distance and/or dissimilarity matrices
15.6 Data standardization
15.7 Standardization, association and dissimilarity
15.8 Multivariate graphics
15.9 Screening multivariate data sets
16 Multivariate analysis of variance and discriminant analysis
16.1 Multivariate analysis of variance (MANOVA)
16.2 Discriminant function analysis
16.3 MANOVA vs discriminant function analysis
16.4 General issues and hints for analysis
17 Principal components and correspondence analysis
17.1 Principal components analysis
17.2 Factor analysis
17.3 Correspondence analysis
17.4 Canonical correlation analysis
17.5 Redundancy analysis
17.6 Canonical correspondence analysis
17.7 Constrained and partial “ordination”
17.8 General issues and hints for analysis
18 Multidimensional scaling and cluster analysis
18.1 Multidimensional scaling
18.2 Classification
18.3 Scaling (ordination) and clustering for biological data
18.4 General issues and hints for analysis
19 Presentation of results
19.1 Presentation of analyses
19.2 Layout of tables
19.3 Displaying summaries of the data
19.4 Error bars
19.5 Oral presentations
19.6 General issues and hints
1.1 Scientific method
1.2 Experiments and other tests
1.3 Data, observations and variables
1.4 Probability
1.5 Probability distributions
2 Estimation
2.1 Samples and populations
2.2 Common parameters and statistics
2.3 Standard errors and confidence intervals for the mean
2.4 Methods for estimating parameters
2.5 Resampling methods for estimation
2.6 Bayesian inference – estimation
3 Hypothesis testing
3.1 Statistical hypothesis testing
3.2 Decision errors
3.3 Other testing methods
3.4 Multiple testing
3.5 Combining results from statistical tests
3.6 Critique of statistical hypothesis testing
3.7 Bayesian hypothesis testing
4 Graphical exploration of data
4.1 Exploratory data analysis
4.2 Analysis with graphs
4.3 Transforming data
4.4 Standardizations
4.5 Outliers
4.6 Censored and missing data
4.7 General issues and hints for analysis
5 Correlation and regression
5.1 Correlation analysis
5.2 Linear models
5.3 Linear regression analysis
5.4 Relationship between regression and correlation
5.5 Smoothing
5.6 Power of tests in correlation and regression
5.7 General issues and hints for analysis
6 Multiple and complex regression
6.1 Multiple linear regression analysis
6.2 Regression trees
6.3 Path analysis and structural equation modeling
6.4 Nonlinear models
6.5 Smoothing and response surfaces
6.6 General issues and hints for analysis
7 Design and power analysis
7.1 Sampling
7.2 Experimental design
7.3 Power analysis
7.4 General issues and hints for analysis
8 Comparing groups or treatments – analysis of variance
8.1 Single factor (one way) designs
8.2 Factor effects
8.3 Assumptions
8.4 ANOVA diagnostics
8.5 Robust ANOVA
8.6 Specific comparisons of means
8.7 Tests for trends
8.8 Testing equality of group variances
8.9 Power of single factor ANOVA
8.10 General issues and hints for analysis
9 Multifactor analysis of variance
9.1 Nested (hierarchical) designs
9.2 Factorial designs
9.3 Pooling in multifactor designs
9.4 Relationship between factorial and nested designs
9.5 General issues and hints for analysis
10 Randomized blocks and simple repeated measures: unreplicated two factor designs
10.1 Unreplicated two factor experimental designs
10.2 Analyzing RCB and RM designs
10.3 Interactions in RCB and RM models
10.4 Assumptions
10.5 Robust RCB and RM analyses
10.6 Specific comparisons
10.7 Efficiency of blocking (to block or not to block?)
10.8 Time as a blocking factor
10.9 Analysis of unbalanced RCB designs
10.10 Power of RCB or simple RM designs
10.11 More complex block designs
10.12 Generalized randomized block designs
10.13 RCB and RM designs and statistical software
10.14 General issues and hints for analysis
11 Split-plot and repeated measures designs: partly nested analyses of variance
11.1 Partly nested designs
11.2 Analyzing partly nested designs
11.3 Assumptions
11.4 Robust partly nested analyses
11.5 Specific comparisons
11.6 Analysis of unbalanced partly nested designs
11.7 Power for partly nested designs
11.8 More complex designs
11.9 Partly nested designs and statistical software
11.10 General issues and hints for analysi
12 Analyses of covariance
12.1 Single factor analysis of covariance (ANCOVA)
12.2 Assumptions of ANCOVA
12.3 Homogeneous slopes
12.4 Robust ANCOVA
12.5 Unequal sample sizes (unbalanced designs)
12.6 Specific comparisons of adjusted means
12.7 More complex designs
12.8 General issues and hints for analysis
13 Generalized linear models and logistic regression
13.1 Generalized linear models
13.2 Logistic regression
13.3 Poisson regression
13.4 Generalized additive models
13.5 Models for correlated data
13.6 General issues and hints for analysis
14 Analyzing frequencies
14.1 Single variable goodness-of-fit tests
14.2 Contingency tables
14.3 Log-linear models
14.4 General issues and hints for analysis
15 Introduction to multivariate analyses
15.1 Multivariate data
15.2 Distributions and associations
15.3 Linear combinations, eigenvectors and eigenvalues
15.4 Multivariate distance and dissimilarity measures
15.5 Comparing distance and/or dissimilarity matrices
15.6 Data standardization
15.7 Standardization, association and dissimilarity
15.8 Multivariate graphics
15.9 Screening multivariate data sets
16 Multivariate analysis of variance and discriminant analysis
16.1 Multivariate analysis of variance (MANOVA)
16.2 Discriminant function analysis
16.3 MANOVA vs discriminant function analysis
16.4 General issues and hints for analysis
17 Principal components and correspondence analysis
17.1 Principal components analysis
17.2 Factor analysis
17.3 Correspondence analysis
17.4 Canonical correlation analysis
17.5 Redundancy analysis
17.6 Canonical correspondence analysis
17.7 Constrained and partial “ordination”
17.8 General issues and hints for analysis
18 Multidimensional scaling and cluster analysis
18.1 Multidimensional scaling
18.2 Classification
18.3 Scaling (ordination) and clustering for biological data
18.4 General issues and hints for analysis
19 Presentation of results
19.1 Presentation of analyses
19.2 Layout of tables
19.3 Displaying summaries of the data
19.4 Error bars
19.5 Oral presentations
19.6 General issues and hints